Deciphering the Hypothesis Test
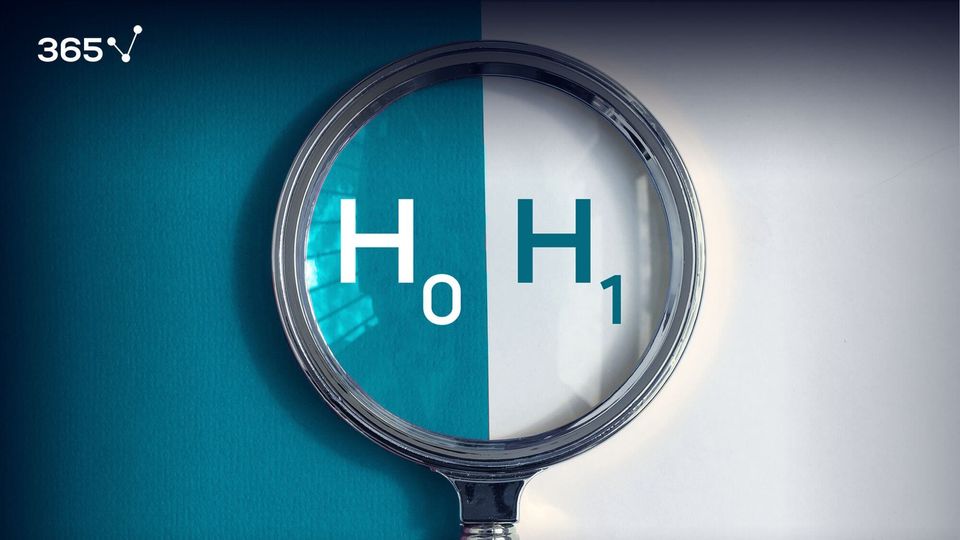
Reference to past blog: In a previous blog, we explained the intuition behind why statistics is a crucial component of experimentation. Today we will attempt to formalize that intuition by introducing the statistical procedure that is used to calculate statistical significance.
Although there have been instances in history of a hypothesis test being conducted as long ago as 1770s (Wikipedia), hypothesis tests were first formalized as the Student's t-test in 1908 (Wikipedia) After almost a quarter-century of being calculated by hand over a maximum of a few 100 samples, the emergence of computing and data gave a boost to Hypothesis Tests which are now being run on datasets of millions. In an introductory statistics course, hypothesis tests are introduced fairly early in the class giving you a strong head buzz that scares away most students.
The Anatomy of a Hypothesis Test sounds complicated because it actually is a twisted answer to a straight-forward question. But the way statistics has had it for 100 years, there was no straight answer to that straight forward question. The straight-forward question was simple, "Looking at the data, what is the probability that they come from two different sources?"
Forward Probability and Backward Probability
There is a fundamental nuance about probability that you need to understand to appreciate the twistedness of a hypothesis test and why it sounds confusing. There are essentially not one but two kinds of probabilities - Forward Probability and Backward Probability. Forward Probability is the probability of an effect given an observed cause. For instance, you might ask what is the chance for one to win a poker hand (Effect) given that they are holding a pair of aces (Cause). I am calling them forward probability because, in the context of time, a cause always precedes the effect. All classical probability was developed to answer questions of forward probability.
There is a fundamentally different kind of probability question that statisticians did not know how to answer for a long time. Backward/Inverse Probability questions were concerned with asking the probability of a cause in retrospection given an observed effect. For instance, if you ask that given that you won a poker hand (Effect), what is the chance that you had initially gotten a pair of aces (Cause). In 1763, Bayes devised the Bayes Rule which gave us a straightforward way to answer backward probability questions. But the subjectivity of the approach and its computational complexity kept it from being used up till the 21st century.
If you carefully observe the core inquiry of a hypothesis test, "Looking at the data (effect), what is the probability that they come from two different sources (cause)?", you will realize that in essence, it is a backward probability question. It is a question that statisticians could not directly solve with the framework of forward probabilities only. Hence, they twisted the answer to our original question.
The Null Hypothesis
To dodge the backward probability question, statisticians looked for a "forward" probability answer to the question of a hypothesis test. They realized that the only easy forward probability calculation could be made under one assumption that both the sources are the same. For any other case, the forward probability calculations demanded that the amount of difference between the two sources should be known which could be anything.
The assumption that both the sources of data do not differ from each other became the Null Hypothesis (H0) in hypothesis testing.
All other possibilities except the null hypothesis became the alternate hypothesis (H1). Finally, a hack was found. If the forward probability of data assuming the null hypothesis came out to be very low, we will reject the null hypothesis and accept the alternate hypothesis. This forward probability of data came to be known as p-values. They were not called the probability of the sources being the same and there has been intense debate in the scientific community on the interpretation of p-values.
P-value is the probability of observing the data that was observed assuming that there is no difference between the two sources of data.
Note that the forward probability from the alternate hypothesis was never calculated in this process and that remains one of the biggest limitations of hypothesis testing. Hypothesis Testing belongs to a broader domain of statistics called the Frequentist Statistics.
Frequentist Statistics solves the problem of hypothesis testing by only taking into account the forward probability. Bayesian Statistics on the other hand uses the backward probability to finally derive a straight answer to the straight-forward question, although it had its own set of problems.
Moving forward
Frequentist and Bayesian Statistics are two forms of the core statistical ideology that have been the source of endless debates, anecdotes, memes, and jokes in statistics. Moving forward, we will dive deep into this dichotomy of statistics and I will tell you the story of their differences, their benefits, and their implications.
However, first, the question still remains how did the Frequentists calculate the forward probability of data assuming that both the sources are the same. To answer simply, they defined a model for a sample taken out from an infinitely large population. This model was the t-distribution that gave us the t-test and the t-statistic. In the next blog, I will take a diversion deeper into statistics to define this t-distribution and explain how it solved the question we were trying to solve.
I recommend the readers who do not want to dive deep into the statistics of the t-test to skim through the next blog and join me back in the discussion between Bayesian and Frequentist Statistics.